Scientists in Korea develop way to classify AAV into 5 patient clusters
Clustering method may be more clinically relevant, researchers say
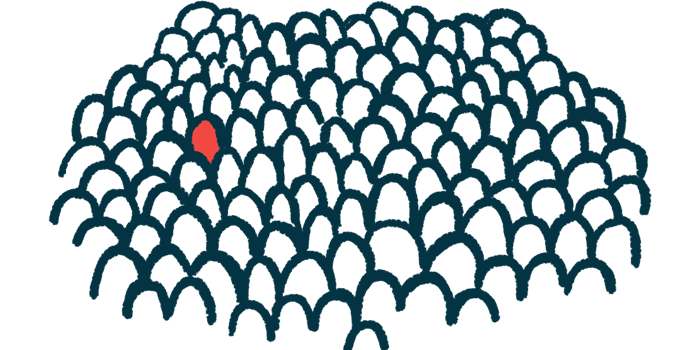
Researchers in Korea have developed a new system to classify ANCA-associated vasculitis (AAV) into clusters, and provided an algorithm that can be used for such classification in future clinical studies.
“This simple algorithm can incorporate any patient with atypical or nonspecific symptoms and can be easily modified to identify patients with a poor prognosis,” scientists wrote in their study “Antineutrophil cytoplasmic antibody-associated vasculitis classification by cluster analysis based on clinical phenotypes: a single-center retrospective cohort study,” which was published in Clinical Rheumatology.
AAV is caused by antibodies that stick to blood cells called anti-neutrophil cytoplasmic autoantibodies (ANCAs) that give the disease its name. The most common ANCAs target either proteinase 3 (PR3) or myeloperoxidase (MPO).
AAV is highly variable, with different experiences for each patient
AAV is a highly variable disease with different experiences for any two people with AAV. From a clinical perspective, this variability can complicate decisions about treatment and make it difficult to make an accurate prognosis.
The disease is divided into subtypes:Â microscopic polyangiitis (MPA), granulomatosis with polyangiitis (GPA), and eosinophilic granulomatosis with polyangiitis (EGPA). However, “overlapping clinical phenotypes of AAV subgroups continually provoke controversies over their diagnostic and classification criteria.”
In this study, a team of scientists at Yonsei University College of Medicine, in South Korea, set out to identify more clinically relevant groupings of AAV.
To do this, the scientists conducted a clustering analysis. Basically, they used sophisticated algorithms to identify groups of patients living with AAV, who were similar based on a certain set of characteristics. Specifically, the analysis included data on age, sex, ANCA status, and AAV severity as measured by the Birmingham Vasculitis Activity Score.
“Even if a patient does not present all the matching phenotypes [characteristics] described in a specific cluster, they can still be a member of the cluster if it is their ‘closest’ cluster,” the researchers wrote. “In other words, a patient is assigned to the most similar cluster based on the clustering variables.”
The analysis used data on 210 people who had been diagnosed with AAV at Yonsei University between 2000 and 2020. Among the patients, 115 had MPA, 53 had GPA, and 42 had EGPA. The median age at diagnosis was 59 years and about a third of the patients were men.
The analysis resulted in five clusters of patients. One cluster, dubbed “generalized PR3-ANCA vasculitis,” included mostly patients positive for ANCAs against PR3, and who had disease affecting many parts of the body. Another cluster, “generalized MPO-ANCA vasculitis,” similarly showed widespread disease involvement throughout the body, but was characterized mostly by patients with ANCAs against MPO.
A third cluster, “limited PR3-ANCA vasculitis,” had mostly patients with ANCAs against PR3 who had symptoms only affecting the ear, nose, and throat. The fourth cluster, “ANCA-negative vasculitis,” included mostly patients who didn’t have detectable ANCAs, while the fifth cluster, “renal-limited vasculitis,” had a high frequency of kidney damage.
No significant differences in all-cause mortality rates between the 5 clusters
Statistical analyses did not show significant differences in all-cause mortality rates between these five clusters, though the researchers noted mortality rates tended to be higher among the two “generalized” clusters. The highest rate of end-stage renal disease was seen in the “renal-limited vasculitis” cluster.
“Based on these results, we proposed a new patient assignment process … to classify patients into one of the five clusters,” the scientists wrote.
They constructed a mathematical algorithm that could be used to assign individual patients into one of these five clusters. They showed it could accurately identify more than 80% of the patients in this analysis.
The team noted that this study was limited to an analysis of patients at a single institution and by a limited follow-up period, so further work is needed to validate the clustering algorithm in broader groups of patients.