AI analysis of clinicians’ notes in medical records helps identify AAV
Machine learning model proves more accurate than traditional algorithms
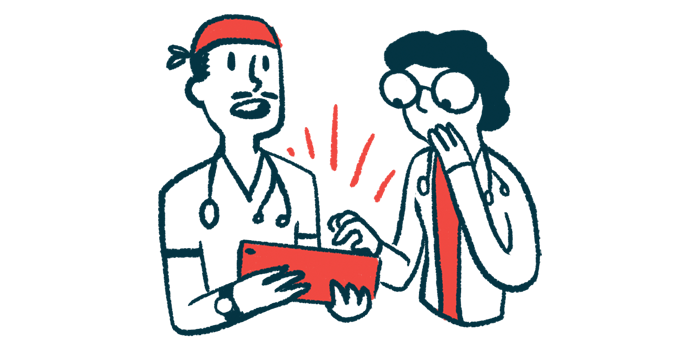
Machine learning based on clinicians’ notes found in electronic health records accurately identifies cases of ANCA-associated vasculitis (AAV), a study demonstrated.
The model was found to be superior to traditional algorithms based on diagnostic codes with or without medication use at identifying AAV cases. “Its application to EHR [electronic health record] notes can potentially uncover additional cases missed by traditional rule-based methods,” the scientists wrote.
The study, “Identification of an ANCA-associated vasculitis cohort using deep learning and electronic health records,” was published in the International Journal of Medical Informatics.
AAV is a rare autoimmune disease in which self-reactive antibodies called ANCAs abnormally activate immune neutrophils, causing damage to small blood vessels. Depending on the tissue or organ affected by blood vessel damage, AAV can manifest in various ways, including problems in the lungs, kidneys, nervous system, and skin.
“AAV case identification in electronic health records (EHR), an increasingly important source for epidemiologic research, is limited by a lack of well-performing methods to identify cases,” the researchers wrote. Epidemiology refers to the study of how often a disease occurs in different groups of people and why.
Mining clinical notes yields ‘rich information’
Available rule-based algorithms for AAV case identification rely on EHR information such as ANCA test results and ICD-9 codes, the codes to assigned diagnoses and procedures in the U.S. Because there is no specific ICD-9 code for microscopic polyangiitis (MPA), a common AAV type, “many MPA patients may be miscoded using less specific ICD-9 codes,” the team wrote.
In addition to ICD-9 codes and test results, EHR data include free text entered by clinicians to document a patient’s diagnosis, symptoms, and related features.
“Clinical notes contain rich information, such as clinical manifestations, physical exams, and differential diagnoses, which are useful to determine whether [a] patient does or does not have AAV,” the researchers wrote.
Research in other diseases has suggested that case-finding algorithms may become more effective when looking at this type of unstructured EHR data. However, whether this is also the case for identifying AAV cases remains unclear.
Scientists at Brigham and Women’s Hospital and Harvard Medical School used machine learning to extract and analyze information from unstructured clinical notes in EHRs.
Machine learning is a form of AI that uses computer algorithms to learn from data and identify and predict patterns.
The team began by collecting data from Mass General Brigham’s research patient registry. This identified a screening cohort of 88,902 potential AAV cases based on at least one AAV-related ICD-9 code or expert-curated keywords in clinical notes.
Three distinct patient groups were then used to develop and evaluate machine-learning algorithms for AAV case identification from clinical notes. Group A comprised 700 people with a confirmed AAV diagnosis, while groups B and C were random samples of 1,000 potential AAV patients.
For each group, clinical notes were extracted and processed into smaller sections. AAV was suggested if any note sections were predicted to be positive, while a patient was deemed non-AAV if all note sections were predicted to be negative.
Testing algorithms
Researchers then created three datasets to test different machine learning algorithms. Dataset 1 contained 5,000 note sections from group A and 1,000 note sections from group B, all mentioning specific keywords.
Dataset 2 contained 2,000 sections from group A and 1,000 from group B that were selected randomly regardless of specific keywords, while dataset 3 consisted of 7,500 random note sections from group C.
After running each dataset through different machine learning models, one model, the hierarchical attention network (HAN) demonstrated the best performance distinguishing AAV from non-AAV cases. Across all three datasets, HAN’s accuracy was greater than 98%.
The team compared the HAN algorithm with two rule-based algorithms using data from group D, which comprised 2,000 individuals randomly selected from the screening cohort.
The first algorithm (Rule 1) was based on AAV-related ICD codes, while the second algorithm (Rule 2) was based on ICD codes and the use of AAV medication within six months.
Using Rule 1, 218 people from group D were identified. After excluding 12 patients due to insufficient information, 40 (19.4%) were confirmed to have AAV. Rule 2 identified 52 patients; 11 (21.2%) had confirmed AAV. A HAN analysis of nearly 2,000 clinical notes predicted AAV in 177 individuals, 45 (26.2%) of whom were confirmed to have AAV.
Overall, the estimated sensitivity, or a method’s ability to correctly identify AAV, was 97.5% for HAN, 87% for Rule 1, and 23.9% for Rule 2.
Machine learning also identified more ANCA-negative AAV cases and patients of Hispanic backgrounds: 24% by HAN versus 2.5% by rule 1 and 0% by rule 2. HAN also detected six additional cases not identified by Rule 1, accounting for 13% of the positive cases, while Rule 1 found one patient not identified by HAN.
“This study is the first to show that a deep learning algorithm can efficiently and accurately identify cases of AAV … in part by only using unstructured EHR data,” the scientists concluded. “This approach has the potential to identify cases that may be overlooked if only using structured EHR data.”