AI model identifies AAV subgroups that better predict outcomes
New patterns could pave way for targeted treatment
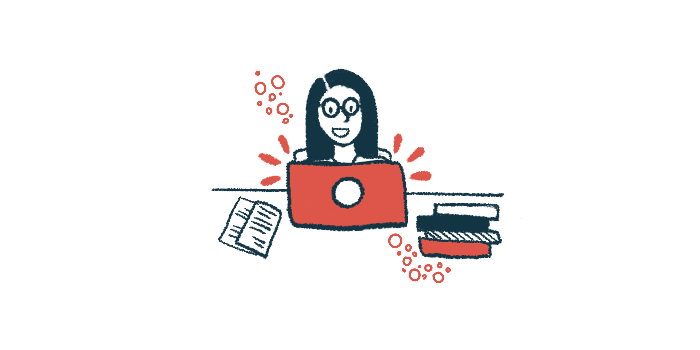
Using data from the largest ANCA-associated vasculitis (AAV) patient database compiled to date, researchers identified five new subgroups that predict patient outcomes better than classifying patients according to granulomatosis with polyangiitis (GPA) and microscopic polyangiitis (MPA), two AAV subtypes.
The new disease clusters, identified with advanced artificial intelligence (AI) and big data techniques, support the use of AI-based technologies to improve the accuracy of patient diagnosis and better tailor therapeutic approaches to each patient’s disease manifestations, the researchers said.
“Our research shows that by leveraging advanced AI systems and broad datasets, we can uncover new patterns of this rare autoimmune disease, which have impacts on the probability of adverse outcomes,” Mark Little, a professor of nephrology at Trinity College Dublin and co-senior author of the study, said in a university news story. “This allows us to focus potentially toxic therapies on those most likely to benefit.”
The study, “Data-driven subclassification of ANCA-associated vasculitis: model-based clustering of a federated international cohort,” was published in The Lancet Rheumatology.
AAV occurs when self-reactive antibodies launch an inflammatory attack against small blood vessels in the body, causing damage to the kidneys and other organs. In AAV, there are two major types of autoantibodies, those targeting the proteinase 3 (PR3) protein and those that target myeloperoxidase (MPO).
Current classifications not enough
Regardless of antibody type, the disease is generally classified into three main types: MPA, GPA, or eosinophilic granulomatosis with polyangiitis (EGPA). While MPA and GPA share some disease characteristics, increasing evidence shows that dividing patients into just these two subtypes is not enough to explain the high disease heterogeneity seen in people with MPA and GPA.
“Both dichotomous subclassifications of ANCA-associated vasculitis, either as GPA and MPA, or PR3-ANCApositive and MPO-ANCA-positive disease, fail to address the considerable heterogeneity seen in disease presentation,” the researchers wrote.
The international research team, led by scientists at Trinity and Sweden’s Lund University, used data from the Findable, Accessible, Interoperable, Reusable, Vasculitis (FAIRVASC) consortium, a collaborative project that in 2023 presented the large database, which includes data from six patient registries in Europe.
In total, the analysis included data from 3,868 AAV patients, with a mean age of 57.2. Men made up 51.9% of the total. About two-thirds (62.9%) had been diagnosed with GPA, and the remaining 37.1% with MPA.
The team used 17 clinical and demographic variables from these patients to build a model that could group patients into different clusters. These included age at diagnosis, gender, levels of serum creatinine and C-reactive protein (markers of liver damage and inflammation) at diagnosis, type of ANCA antibodies, and the presence of certain symptoms.
The analysis identified five new disease subgroups with distinct manifestations and outcomes. Three subgroups were characterized by kidney involvement. One subgroup had severe kidney disease with high creatinine and C-reactive protein levels, one was positive for anti-MPO antibodies and had limited symptoms outside the kidneys, and the third had anti-PR3 antibodies and extensive manifestations outside the kidney.
The other two groups were marked by a relative absence of kidney symptoms. One had predominantly anti-PR3 antibodies and inflammation across the body, while the other included mainly younger patients, with low C-reactive protein levels and symptoms involving the ear, nose, and throat.
This last cluster had the best prognosis in terms of survival and kidney failure, with only 5.6% and 1.7% of patients reaching those endpoints over a median follow-up of 4.2 years. In turn, the severe kidney disease group had the worst prognosis, with 30.5% dying and 41.6% reaching kidney failure over the follow-up period.
According to the researchers, the new AI-driven model showed a better predictive power regarding both survival and kidney outcomes when compared with traditional classification models.
“Such progress was possible only through a multidisciplinary approach and with direct involvement of patients with lived experience of the condition, and this collaborative project has successfully brought together experts in medicine, computer science, and statistics,” Little said.